Jiaxuan Guo
Fudan University. 220 Handan Rd, Shanghai.
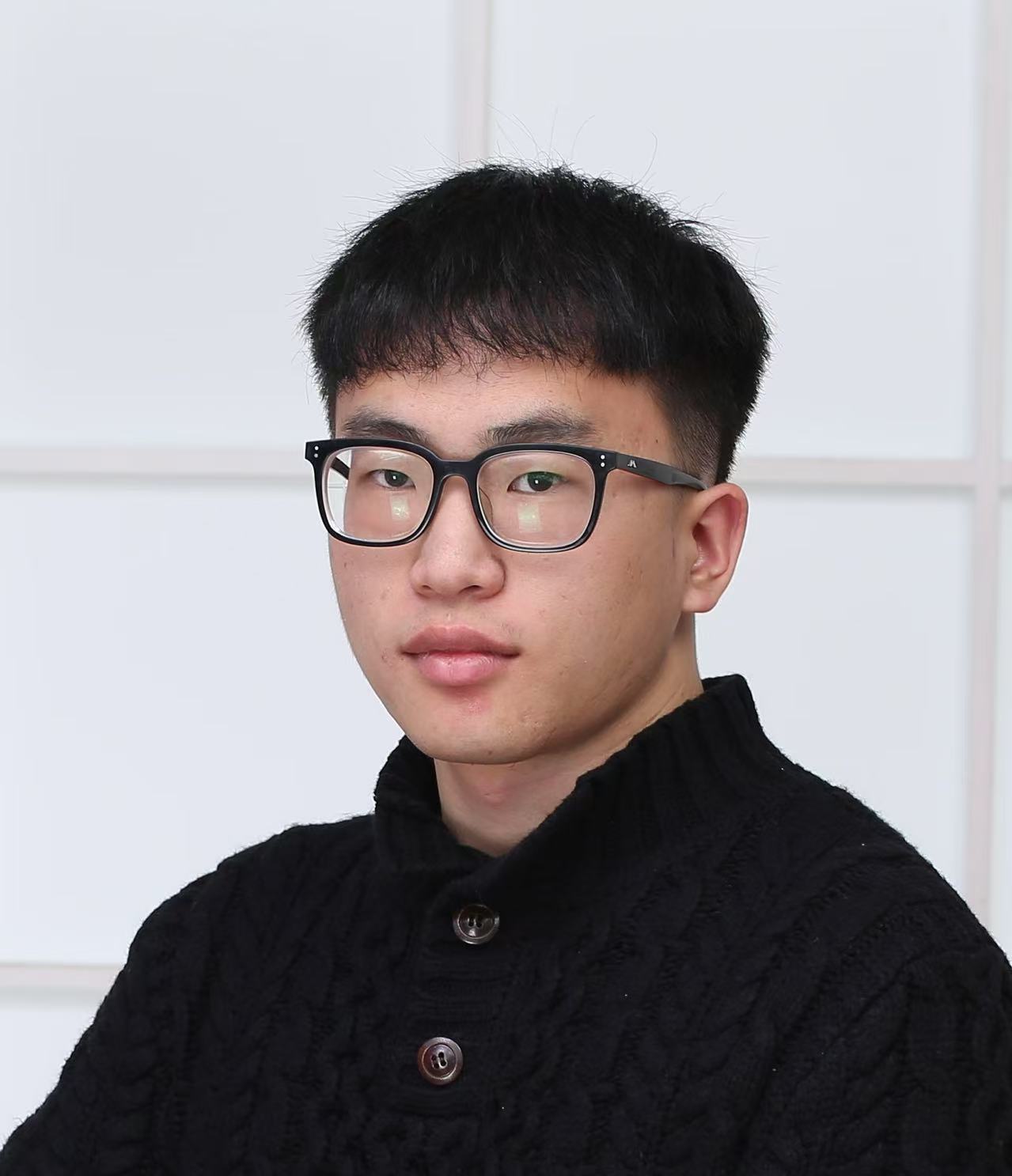
Email:jiaxuanguo20@gmail.com
Phone:(+86)187-3604-1701
Hi there! I am Jiaxuan Guo, a fourth-year student majoring in Physics at Fudan University in China. My research interests lie in computational physics and materials science, especially by means of first-principles calculations and machine learning.
Under the guidance of Prof. Jing Wang at Fudan University, I conducted research on the utilization of deep learning techniques for the discovery of topological and superconducting materials. Additionally, I worked under the supervision of Prof. Diana Qiu at Yale University, where I employed first-principles calculations to investigate the behavior of excitons in 2D twisted heterostructures.
I am applying for 2024 fall Ph.D. in physics and materials science!
Research Topics
- Ab Initio Methods for Electronic and Optical Properties of Real Materials
- Twisted 2D Bilayer Materials
- Artificial Intelligence for Science (Physics, Material Science)
Experience
(Details seen in CV)
First-Principles Study of Time-Resolved ARPES on t-MoSe2/WS2 Bilayer
Supervised by Prof. Diana Qiu
May 2023 – Present Yale University
Part I: Density Functional Theory (DFT) Level Study
- Employed the Quantum ESPRESSO package to analyze electronic orbital-projected band structures, wavefunctions, and density of states (DOS) of the twisted MoSe2/WS2 heterostructure.
- Developed a theoretical model to predict the oscillation period resulting from the interference between electrons in the top and bottom layers.
- Computed the K point matrix elements in ARPES via the free electron approximation, thereby simulating the photoemission (PE) intensity and validating the theoretical model.
Part II: Exciton Level Study
- Utilized the BerkeleyGW package to perform a one-shot G0W0 calculation and applied the Bethe-Salpeter equation (BSE) approach to characterize excitonic properties, including exciton binding energy and exciton wavefunction.
- Developed scripts to visualize the distribution of excitons within momentum space and computed the band-to-band transition matrix term (no free electron approximation) at each k-point.
- Simulated the PE intensity from the exciton level and put forward an explanation for the oscillations observed in the experiment
- Discovered discrepancies between experimental results and literature regarding the band alignment, challenging the computational accuracy of prior research on MoSe2/WS2 heterostructure.
Deep Learning Approach to Novel Topological Materials
Supervised by Prof. Jing Wang
Dec. 2022 – Apr. 2023 Fudan University
- Learned some machine learning (ML) methods, including Convolutional Neural Network (CNN), Support Vector Machine (SVM), HDBSCAN, and t-SNE.
- Applied CNN algorithms to streamline the exploration of materials exhibiting notably clean Fermi surfaces, achieving an accuracy rate exceeding 90%.
- Identified approximately 150 prospective material candidates from a pool of 2,000 entries within the 2Dmatpedia database, significantly accelerating the process of seeking novel topological materials.
Crystal Graph Convolutional Neural Networks for Prediction of Superconductors
Supervised by Prof. Jing Wang
Sept. 2023 – Present Fudan University
- Constructed a training dataset comprising 1120 CIF structure files of superconducting materials along with their corresponding transition temperatures Tc , and this dataset is continuously expanding.
- Based on Atomistic Line Graph Neural Network (ALIGNN), currently enhancing the input node features and model framework to improve prediction accuracy.